Managing COVID-19 outbreaks with screening & surveillance
Viruses like the one responsible for COVID-19—known as the SARS-CoV-2 virus—are particularly good at spreading throughout a community. Not only can this virus be spread before symptoms develop, at any given moment, approximately 50-90% of individuals with SARS-CoV-2 have yet to develop symptoms (and may never do so)1-6.
An infected individual may be contagious for more than a week following infection3-6. This means that in the absence of public safety measures, it’s likely that one infected individual will quickly give rise to many more.
Fortunately, there are ways to limit the spread of viruses like SARS-CoV-2. Social distancing measures, face mask wearing, and handwashing, for example, have been encouraged in nations around the world7-10. However, as much of the world prepares to return to work, such measures can’t prevent viral spread on their own.
To prevent outbreaks, we as a society—from healthcare systems and governments to schools and small businesses—will need the ability to:
- Quickly identify people who are infected with SARS-CoV-2;
- Help those individuals properly quarantine while receiving aid; and
- Make adjustments based on infection rate statistics.
In short, we need the ability to perform what’s known as screening and surveillance testing.
Screening for COVID-19 involves broad-scale testing of a population to see who may be infected with SARS-CoV-2. This type of testing can help identify infected individuals before they’ve had a chance to spread the virus to many others. And, if infected individuals quickly undergo a temporary quarantine, use of screening can decrease the likelihood of large COVID-19 outbreaks11.
Surveillance testing is slightly different. As its name suggests, surveillance testing aims to surveil a population at a high level using data—such as the percentage of people who have tested positive in a population—to identify and respond to outbreaks. In practice, this would involve recurring testing of individuals from a population to see if and how the rate of infection is changing overtime. If the rate of infection increases, it suggests an outbreak has started and that adjustments should be made in public health measures to curtail it.
In the sections below, we describe how the use of COVID-19 screening and the combined use of screening results for surveillance can be an effective way to manage COVID-19 outbreaks under various conditions based on epidemiological modeling. (You can find our white paper describing this data in more detail here.)
It’s important to remember that modeling requires selecting parameters for many variables, which may be different from reality. The results below should be taken with this in mind and thought of as an estimation which may differ from results reported in models that use different parameters.
Test turn around time
It’s worth noting that the success of screening and surveillance testing is largely dependent on the availability of sensitive COVID-19 tests with a rapid turnaround time (within 24 hours of testing)14.
Longer turnaround times for test results lead to a longer window in which infectious individuals can spread the virus to others. Further, if there is a long lag between testing and results, quarantine of infected individuals will be far less effective at reducing spread of infection.
Considerations when selecting a testing system are beyond the scope of this article and a rapid turnaround time is assumed for all analyses below.
Measuring success with the reproductive number (R0)
It’s difficult to know exactly how contagious a virus like SARS-CoV-2 is, but some estimates suggest that without any public health measures, one person infected with SARS-CoV-2 may infect as many as six other people12. To put it another way, under certain conditions, the SARS-CoV-2 virus has an R0 value of 6.
R0 represents the number of people that a single infected person is likely to spread the virus to over a designated amount of time13. It can be thought of as a measurement of how rapidly a virus is likely to spread under specific conditions.
In order to prevent outbreaks of COVID-19 when re-opening society, we’ll need to control the R0 value to keep it at or below 1—meaning that an infected person will likely only spread the virus to one other person.
Our modeling suggests that COVID-19 screening and then use of the screening data for surveillance can be an effective way to do that.
Modeling results for various screening testing frequencies
As mentioned above, communities can influence the R0 value for a virus depending on what type of public health measures are in place, how strictly people adhere to those measures, and how quickly and effectively we can identify and quarantine people who are infected.
This can be done, in part, by screening people with COVID-19 tests and using that data to surveil for outbreaks.
We modeled how different screening frequencies—how often you test people within a population—would impact the spread of the virus when there are strict public health measures as well as when there aren’t.
This model is known as an SIR model, which stands for Susceptible, Infectious, and Recovered—the three general states a person can be in during a pandemic caused by a novel pathogen. In our model, we estimated that 80% of the people who test positive would adhere to safety measures because it’s very unlikely that every person in a population would follow the established guidelines perfectly.
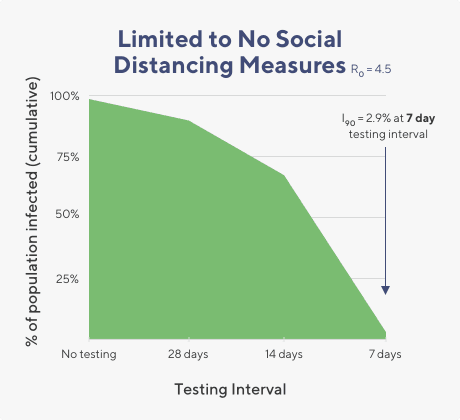
We found that when the R0 value is ~4.5 (similar to what the CDC estimates would be the R0 value when there is widespread community transmission) that testing every person within a set population once a week can help to bring the rate of infection down to below 3% total infections over 90 days.
If testing in this scenario is limited to just once a month, the infection rate remains high (near 85% infected) after 90 days.
In short, when the rate of community spread is high, screening would need to be once weekly to keep infection rates low.
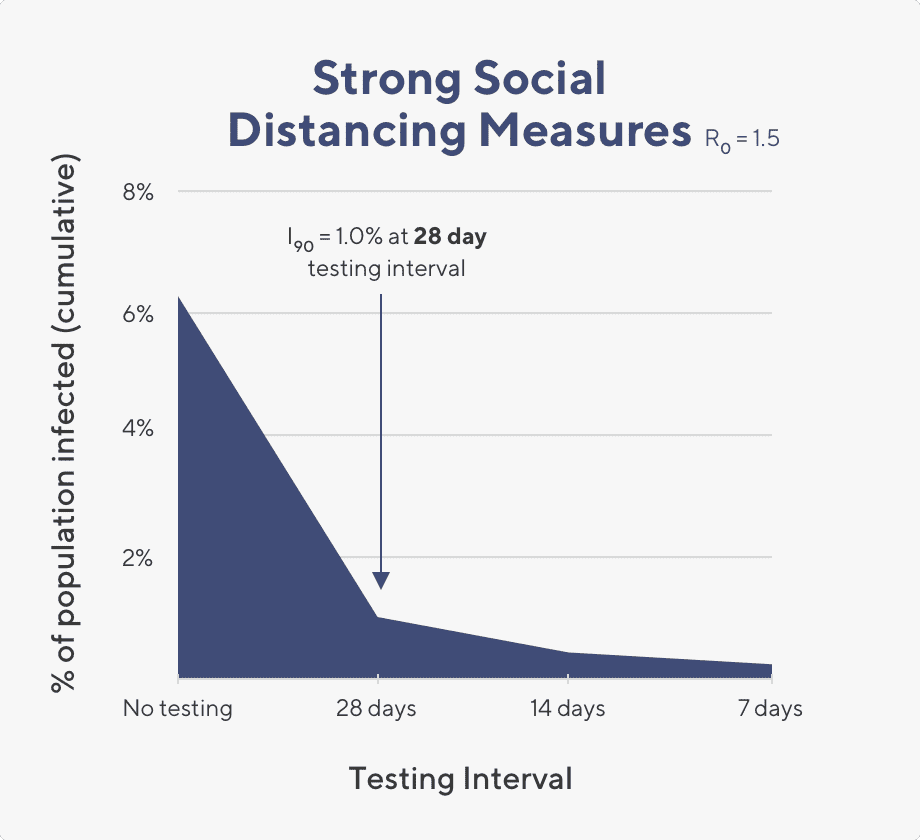
Graphic showing model results when R0 is 1.5. I90 indicates what the percentage of infected individuals would be within 90 days under the stated conditions.
In contrast, when the rate of community transmission is low and the R0 value is about 1.5, a testing cadence of once a month works well to reduce the infection rate down below 2% total infections within that same time frame. Here, screening for COVID-19 can be relaxed so that once a month testing is sufficient to keep outbreaks at bay.
What we see in these two cases is that testing frequency can be modified based on the R0 value. However, in real time, it is difficult to know exactly what the R0 value is, especially if you’re not a statistician. But what you can do is monitor for spikes in infections. If you see a sudden spike above the infection rate threshold you have set as acceptable (1%, for example) then you know there is a likelihood that your R0 value has increased. Why it increased will be hard to know, but it’s possible that people are not following public health measures as strictly as they should. As we saw above, testing cadence can be shortened (to one week, for example) to help re-establish a safe environment.
Taken together, these results suggest that frequent testing can help reduce the spread of SARS-CoV-2. However, the exact frequency of testing needed depends on several factors. If one of these factors changes—such as a relaxation of public health measures—it would be important to re-evaluate, and likely increase testing frequency.
Example of screening and surveillance testing in action ▼
Screening and surveillance testing can help you identify outbreaks before they have a chance to grow. Testing cadence can be modified to respond to outbreaks: if the number of infected individuals increases, an increased testing cadence can help curtail the outbreak. Below is a theoretical example of how screening and surveillance testing can be used to maintain a low infection rate in the workplace.
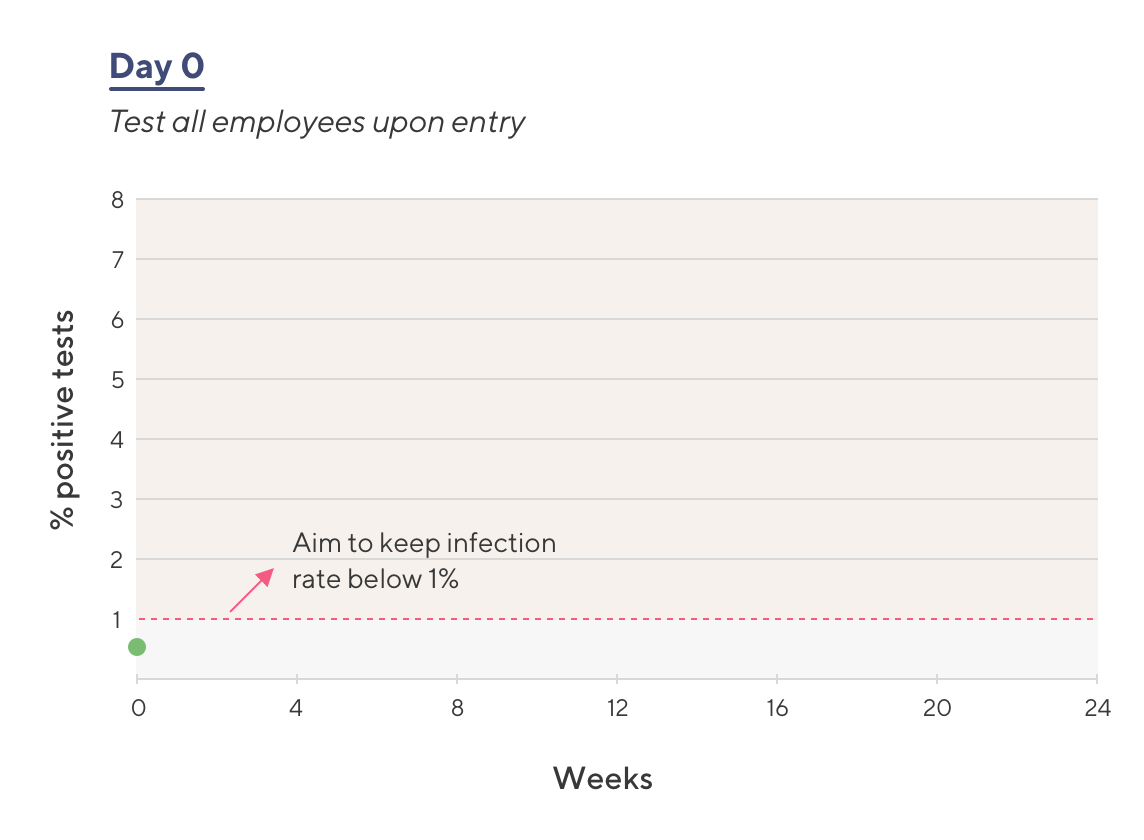
A 1,000 person company is looking to launch testing for non-essential employees.
- Employees have been self quarantined for the past few months
- Upon returning to the office, employees will be required to wear masks
Estimated R0 on the first day back to work: 1.5
- All employees are screened on the first day; fewer than 1% of employees are infected
- All employees are again screened one week after coming back to work; fewer than 1% of employees are infected
- Three weeks after coming back to the work, all employees are again screened; fewer than 1% of employees are infected
Based on low rate of infection, ACME Health leadership switches to a once-monthly testing cadence
- Seven weeks after returning to work, employees are again tested; fewer than 1% of employees are infected
- Eleven weeks after returning to work, employees are tested; this time, almost 2.5% of employees test positive for SARS-CoV-2
The spike in positive cases suggests that something has changed causing the R0 value to increase
- Testing cadence is increased to once weekly.
- After 2 months, the percentage of infected employees decreases below the 1% threshold
The low percentage of infections enables ACME leadership to decrease testing cadence back to once monthly
Limitations and important considerations
For simplicity, our models assumed that populations are isolated, meaning people did not leave or enter the population. However, in reality, we may expect all populations to interact, in some form, with the broader community. These interactions may lead to the continuous reintroduction of SARS-CoV-2 into your population.
Screening and surveillance testing can still be effective in controlling outbreaks even with frequent introduction of infected individuals into your population: if the number of people entering a population are expected to be high, outbreaks can be suppressed by testing at a higher frequency than what would be required in an isolated population.
Otherwise, if the rate of transmission from the broader community is expected to be low, the model described above offers a reasonable prediction of infection dynamics.
Our model is also limited in its assumptions that all positive cases are detected and isolated via screening. However, it is likely that in the period between screenings, diagnostic testing would be provided for symptomatic individuals to diagnose and quarantine infections as they arise. These actions will lower the level of community infection predicted by the surveillance-testing-only scenario and, in effect, allow for longer time intervals between surveillance tests.
It is likely that different subsets of your community have different levels of risk of infection. For example, some parts of your population may be involved in congregate settings where social distancing is not possible. Stratifying testing frequency based on risk may lead to better, targeted, testing; however the trade-off is a more complex screening and surveillance testing schedule. Additionally, the starting R0 value is difficult to determine. The true R0 for SARS-CoV-2 in each community will differ and may be difficult to estimate before testing data has been collected over a reasonable time period.
Conclusions
Our model suggests that screening, combined with surveillance testing, in the form of weekly testing makes it possible to rapidly detect and prevent would-be outbreaks of COVID-19, even under conditions where public safety measures are more relaxed. With social distancing and other safety measures in place, outbreaks can be contained with less frequent testing.
We propose that outbreaks can be contained by determining a threshold for the acceptable percentage of people in a population that are infected (such as 1-2%). With this, week-to-week infection rates can be measured and if the threshold is passed, testing frequency can be increased to contain the outbreak (see Box 1, for an example scenario)
In populations with regular community reintroduction and minimal social distancing, weekly testing will likely be required. If social distancing measures are adopted, and community transmission drops, then testing can be reduced to biweekly or monthly.
In all scenarios, if positive tests exceed the target threshold for positive cases during a given window, testing should return to a weekly testing cadence to reestablish control until the percentage of infected individuals in the population stabilizes.
References
1. Oran, Daniel P., and Eric J. Topol. 2020. “Prevalence of Asymptomatic SARS-CoV-2 Infection: A Narrative Review.” Annals of Internal Medicine, June. https://doi.org/10.7326/M20-3012.
2. Ferretti, Luca, Chris Wymant, Michelle Kendall, Lele Zhao, Anel Nurtay, Lucie Abeler-Dörner, Michael Parker, David Bonsall, and Christophe Fraser. 2020. “Quantifying SARS-CoV-2 Transmission Suggests Epidemic Control with Digital Contact Tracing.” Science 368 (6491). https://doi.org/10.1126/science.abb6936.
3. He, Xi, Eric H. Y. Lau, Peng Wu, Xilong Deng, Jian Wang, Xinxin Hao, Yiu Chung Lau, et al. 2020. “Temporal Dynamics in Viral Shedding and Transmissibility of COVID-19.” Nature Medicine 26 (5): 672–75.
4. Li, Ruiyun, Sen Pei, Bin Chen, Yimeng Song, Tao Zhang, Wan Yang, and Jeffrey Shaman. 2020. “Substantial Undocumented Infection Facilitates the Rapid Dissemination of Novel Coronavirus (SARS-CoV-2).” Science 368 (6490): 489–93.
5. Arons, Melissa M., Kelly M. Hatfield, Sujan C. Reddy, Anne Kimball, Allison James, Jesica R. Jacobs, Joanne Taylor, et al. 2020. “Presymptomatic SARS-CoV-2 Infections and Transmission in a Skilled Nursing Facility.” The New England Journal of Medicine 382 (22): 2081–90.
6. Lauer, Stephen A., Kyra H. Grantz, Qifang Bi, Forrest K. Jones, Qulu Zheng, Hannah R. Meredith, Andrew S. Azman, Nicholas G. Reich, and Justin Lessler. 2020. “The Incubation Period of Coronavirus Disease 2019 (COVID-19) From Publicly Reported Confirmed Cases: Estimation and Application.” Annals of Internal Medicine 172 (9): 577–82.
7. Doung-ngern, Pawinee, et al. 2020 “Associations between wearing masks, washing hands, and social distancing practices, and risk of COVID-19 infection in public: a cohort-based case-control study in Thailand.” medRxiv
8. Teslya, Alexandra, et al. “Impact of Self-Imposed Prevention Measures and Short-Term Government-Imposed Social Distancing on Mitigating and Delaying a COVID-19 Epidemic: A Modelling Study.” PLOS Medicine, vol. 17, no. 7, 2020, doi:10.1371/journal.pmed.1003166.
9. Qian, Meirui, and Jianli Jiang. “COVID-19 and social distancing.” Zeitschrift Fur Gesundheitswissenschaften, 1–3. 25 May. 2020, doi:10.1007/s10389-020-01321-z
10. “Advice for the Public on COVID-19.” World Health Organization, World Health Organization, www.who.int/emergencies/diseases/novel-coronavirus-2019/advice-for-public.
11. Taipale, Jussi, Paul Romer, and Sten Linnarsson. 2020. “Population-Scale Testing Can Suppress the Spread of COVID-19.” Epidemiology. medRxiv. https://doi.org/10.1101/2020.04.27.20078329.
12. Steven Sanche, Yen Ting Lin, Chonggang Xu, Ethan Romero-Severson, Nick Hengartner, and Ruian Ke. 2020. “High Contagiousness and Rapid Spread of Severe Acute Respiratory Syndrome Coronavirus 2.” Emerging Infectious Disease Journal 26 (7). https://doi.org/10.3201/eid2607.200282.
13. Diekmann, O., J. A. Heesterbeek, and J. A. Metz. 1990. “On the Definition and the Computation of the Basic Reproduction Ratio R0 in Models for Infectious Diseases in Heterogeneous Populations.” Journal of Mathematical Biology 28 (4): 365–82.
14. Larremore DB, Wilder B, Lester E, Shehata S, Burke JM, Hay JA, et al. Test sensitivity is secondary to frequency and turnaround time for COVID-19 surveillance. Infectious Diseases (except HIV/AIDS). medRxiv; 2020. doi:10.1101/2020.06.22.20136309
16. Peto J, Carpenter J, Smith GD, Duffy S, Houlston R, Hunter DJ, et al. Weekly COVID-19 testing with household quarantine and contact tracing is feasible and would probably end the epidemic. Royal Society Open Science. 7: 200915.
17.Paul L. Delamater, Erica J. Street, Timothy F. Leslie, Y. Tony Yang, and Kathryn H. Jacobsen. 2019. “Complexity of the Basic Reproduction Number (R0).” Emerging Infectious Disease Journal 25 (1): 1
Categories