How do scientists find new insights in the human genome?
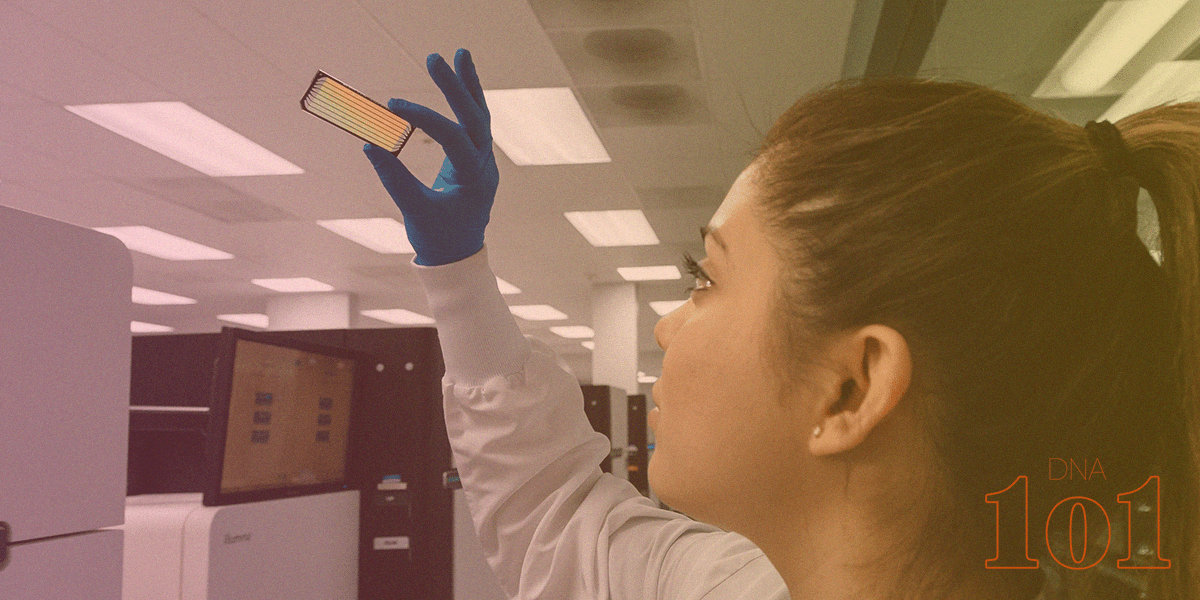
Scientists around the world have exponentially increased our knowledge in the field of genetics over the last decade by uncovering a huge number of genetic insights. Many of these insights have come from large-scale endeavors called genome-wide association studies (GWAS). GWAS is an experimental design—that is, a way that experiments are structured and carried out—that involves searching the genomes of large numbers of people for common small variants in DNA that are associated with a particular trait. These studies are based on the idea that the associated variants will be more commonly found in individuals who have the trait than in those who do not. GWAS have been used to find associations with disease related traits such as asthma, diabetes, and heart disease, and with non-disease related traits, such as height and hair color.
GWAS are useful in studying common, complex traits in which many genes and environmental factors may contribute to the trait (as opposed to traits that are entirely determined by an individual’s genetic makeup, such as Huntington’s Disease1). A good example of a complex trait is height. When we look at the overall distribution of height across a population, we see a range of heights, not just “short” or “tall.” Height is a polygenic trait, meaning that it is determined by the interaction of multiple genes. There are many different genetic variations that contribute to a person’s height and each of these variants has its own small effect. Some variants might be associated with increased height, while others might be associated with decreased height. It is the interaction between all of these variants, in addition to environmental factors, that results in an individual’s actual height.
In order to determine which variants are associated with a trait, researchers first recruit individuals with the trait that they are interested in studying (cases) and individuals who do not have the trait (controls). They collect DNA from each participant and then scour the genomes of these individuals for variants that occur more frequently in one of these groups than in the other. Statistical analysis is then performed to determine whether the variants are significantly associated with the trait.
GWAS studies are often seen as being “hypothesis-generating”
GWAS studies are often seen as being “hypothesis-generating,” because they identify which variants are associated with the trait by highlighting the regions of the genome that are likely to be important to its biology—but the studies themselves do not explain that underlying biology. The variations found in GWAS studies may not directly cause the trait; they might just be associated with the causal variants, which is why they are turning up as hits in the analysis. Therefore, the variants that are found to be significant hits in a GWAS are then selected for follow-up functional studies to determine if and how they are actually causing the trait.
As we move forward in the field of genetic research, GWAS have continued to evolve in order to provide more useful insights to researchers. With larger sample sizes that now include more individuals from non-European populations, researchers hope to identify more genes that might have smaller effect sizes or are more prevalent in certain populations.
GWAS studies have helped us in our journey of uncovering the biological underpinnings of both disease-related and non-disease related traits. Studies have led to the discovery of the genetic underpinnings of diseases such as schizophrenia, type 2 diabetes, and certain autoimmune diseases. These kinds of discoveries can, in turn, be used to develop better diagnostic tests and therapeutics. We still have a long road ahead of us when it comes to understanding the underlying biology of many traits, but GWAS studies have helped provide us with the first step in the discovery process.
1Visscher, Peter M. et al. “10 Years of GWAS Discovery: Biology, Function, and Translation.” American Journal of Human Genetics 101.1 (2017): 5–22. PMC. Web. 19 June 2018.